real-time analytics
What is real-time analytics?
Real-time analytics is the use of data and related resources for analysis as soon as it enters the system. The adjective real-time refers to a level of computer responsiveness that a user senses as immediate or nearly immediate. The term is often associated with streaming data architectures and real-time operational decisions that can be made automatically through robotic process automation and policy enforcement.
Whereas historical data analysis uses a set of historical data for batch analysis, real-time analytics instead visualizes and analyzes the data as it appears in the computer system. This enables data scientists to use real-time analytics for the following purposes:
- forming operational decisions and applying them to production activities -- including business processes and transactions -- on an ongoing basis;
- viewing dashboard displays in real time with constantly updated transactional data sets;
- utilizing existing prescriptive and predictive analytics; and
- reporting historical and current data simultaneously.
Real-time analytics software has the following basic components:
- an aggregator that gathers data event streams -- and perhaps batch files -- from a variety of data sources;
- a broker that makes data available for consumption; and
- an analytics engine that analyzes the data, correlates values and blends streams together.
The system that receives and sends data streams and executes the application and real-time analytics logic is called the stream processor.
How real-time analytics works
Real-time analytics often takes place at the edge of the network to ensure that data analysis is done as close to the data's origin as possible. In addition to edge computing, other technologies that support real-time analytics include the following:
- Processing in memory is a chip architecture in which the processor is integrated into a memory chip to reduce latency.
- In-database analytics is a technology that allows data processing to be conducted within the database by building analytic logic into the database itself.
- Data warehouse appliances are a combination of hardware and software products designed specifically for analytical processing. An appliance allows the purchaser to deploy a high-performance data warehouse right out of the box.
- In-memory analytics is an approach to querying data when it resides in random access memory, as opposed to querying data that is stored on physical disks.
- Massively parallel programming is the coordinated processing of a program by multiple processors that work on different parts of the program, with each processor using its own operating system and memory.
In order for the real-time data to be useful, the real-time analytics applications being used should have high availability and low response times. These applications should also feasibly manage large amounts of data, up to terabytes. This should all be done while returning answers to queries within seconds.
The term real-time also includes managing changing data sources -- something that may arise as market and business factors change within a company. As a result, the real-time analytics applications should be able to handle big data.
The adoption of real-time big data analytics can do the following:
- maximize business returns;
- reduce operational costs; and
- introduce an era where machines can interact over the internet of things using real-time information to make decisions on their own.
Different technologies exist that have been designed to meet these demands, including the growing quantities and diversity of data. Some of these new technologies are based on specialized appliances -- such as hardware and software systems. Other technologies utilize a special processor and memory chip combination, or a database with analytics capabilities embedded in its design.
Benefits of real-time analytics
Real-time analytics enables businesses to react without delay, quickly detect and respond to patterns in user behavior, take advantage of opportunities that could otherwise be missed and prevent problems before they arise.
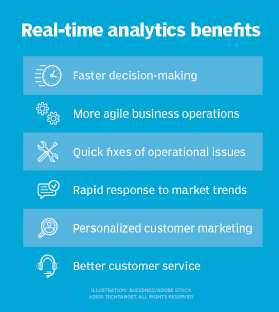
Businesses that utilize real-time analytics greatly reduce risk throughout their company. The system uses data to predict outcomes and suggest alternatives rather than relying on the collection of speculations based on past events or recent scans -- as is the case with historical data analytics. Real-time analytics provides insights into what is going on in the moment.
Other benefits of real-time analytics include the following:
- Data visualization. Real-time data can be visualized and reflects occurrences throughout the company as they occur, whereas historical data can only be placed into a chart in order to communicate an overall idea.
- Improved competitiveness. Businesses that use real-time analytics can identify trends and benchmarks faster than their competitors who are still using historical data. Real-time analytics also allows businesses to evaluate their partners' and competitors' performance reports instantaneously.
- Precise information. Real-time analytics focuses on instant analyses that are consistently useful in the creation of focused outcomes, helping ensure time is not wasted on the collection of useless data.
- Lower costs. While real-time technologies can be expensive, their multiple and constant benefits make them more profitable when used long term. Furthermore, the technologies help avoid delays in using resources or receiving information.
- Faster results. The ability to instantly classify raw data allows queries to more efficiently collect the appropriate data and sort through it quickly. This, in turn, allows for faster and more efficient trend prediction and decision making.
Challenges of real-time analytics
Real-time analytics challenges can include how to define the term, create an architecture, change business processes and train employees.
1. How it's defined
One major challenge faced in real-time analytics is the vague definition of real time and the inconsistent requirements that result from the various interpretations of the term. As a result, businesses must invest a significant amount of time and effort to collect specific and detailed requirements from all stakeholders in order to agree on a specific definition of real time, what is needed for it and what data sources should be used.
2. Required system architecture
Once the company has unanimously decided on what real time means, it faces the challenge of creating an architecture with the ability to process data at high speeds. Unfortunately, data sources and applications can cause processing-speed requirements to vary from milliseconds to minutes, making creation of a capable architecture difficult. Furthermore, the architecture must also be capable of handling quick changes in data volume and should be able to scale up as the data grows.
3. Business process changes
The implementation of a real-time analytics system can also present a challenge to a business's internal processes. The technical tasks required to set up real-time analytics -- such as creation of the architecture -- often cause businesses to ignore changes that should be made to internal processes. Enterprises should view real-time analytics as a tool and starting point for improving internal processes rather than as the ultimate goal of the business.
4. Employee training
Finally, companies may find that their employees are resistant to the change when implementing real-time analytics. Therefore, businesses should focus on preparing their staff by providing appropriate training and fully communicating the reasons for the change to real-time analytics.
Real-time analytics use cases in customer experience management
In customer relationship management and customer experience management, real-time analytics can provide up-to-the-minute information about an enterprise's customers and present it so that better and quicker business decisions can be made -- perhaps even within the time span of a customer interaction.
The following examples demonstrate how organizations can use real-time analytics:
- Fine-tuning features for customer-facing apps. Real-time analytics adds a level of sophistication to software rollouts and supports data-driven decisions for core feature management.
- Managing location data. Real-time analytics can be used to determine what data sets are relevant to a particular geographic location and signal the appropriate updates.
- Detecting anomalies and frauds. Real-time analytics can be used to identify statistical outliers caused by security breaches, network outages or machine failures.
- Empowering advertising and marketing campaigns. Data gathered from ad inventory, web visits, demographics and customer behavior can be analyzed in real time to uncover insights that hopefully will improve audience targeting, pricing strategies and conversion rates.
Real-time analytics examples
Examples of real-time analytics include the following:
- Real-time credit scoring. Instant updates of individuals' credit scores allow financial institutions to immediately decide whether or not to extend the customer's credit.
- Financial trading. Real-time big data analytics is being used to support decision-making in financial trading. Institutions use financial databases, satellite weather stations and social media to instantaneously inform buying and selling decisions.
- Targeting promotions. Businesses can use real-time analytics to deliver promotions and incentives to customers while they are in the store and surrounded by the merchandise to increase the chances of a sale.
- Healthcare services. Real-time analytics is used in wearable devices -- such as smartwatches -- and has already proven to save lives through the ability to monitor statistics, such as heart rate, in real time.
- Emergency and humanitarian services. By attaching real-time analytical engines to edge devices -- such as drones -- incident responders can combine powerful information, including traffic, weather and geospatial data, to make better informed and more efficient decisions that can improve their abilities to respond to emergencies and other events.
What's the future of real-time analytics?
The future of pharmaceutical marketing and sales is being greatly impacted by the use of real-time analytics. It is expected that more pharmaceutical companies will begin using emerging technologies and implementing real-time analytics instead of relying on traditional methods to gain deeper insights into customer behavior and the market landscape. This has the potential to reduce costs through accurate predictions while also increasing sales and profit by optimizing marketing.
Higher education is also changing with the use of real-time analytics. Organizations can start marketing to prospective students who are best fit for their institution based on factors such as test scores, academic records and financial standing. Real-time, predictive analytics can help educational organizations gauge the probability of the student graduating and using their degree for gainful employment as well as predict a class' debt load and earnings after graduation.
Unfortunately, the consistently increasing amount of machines and technical devices in the world and the expanding amount of information they capture makes it harder and harder to gain valuable insights from the data. One solution to this is the open source Elastic stack; a collection of products that centralizes, stores, analyzes and displays any desired log and machine data in real time. Open Source is believed to be the future of computer programs, especially in data-driven fields like business intelligence.
Editor's note: This article was written by Kate Brush in 2019. TechTarget editors revised it in 2022 to improve the reader experience.